GMS: Grid-based Motion Statistics for Fast, Ultra-robust Feature Correspondence
Abstract
Incorporating smoothness constraints into feature matching is known to enable ultra-robust matching. However, such formulations are both complex and slow, making them unsuitable for video applications. This paper proposes GMS (Grid-based Motion Statistics), a simple means of encapsulating motion smoothness as the statistical likelihood of a certain number of matches in a region. GMS enables translation of high match numbers into high match quality. This provides a real-time, ultra-robust correspondence system. Evaluation on videos, with low textures, blurs and wide-baselines show GMS consistently out-performs other real-time matchers and can achieve parity with more sophisticated, much slower techniques.
Publication
Other Resources
The paper was selected and reviewed by Computer Vision News.
The method has been integrated into OpenCV library (see function “matchGMS” in [opencv_contrib/xfeatures2d]).
More experiments are shown in MatchBench.
Core idea: Motion Statistics
Observation: True correspondences normally appear certain consistency in term of the motion while false correspondences do not. Hence, we predict that a true match may have many more supporting matches in its surrounding area than a false match.
Partionability: Assign the motion statistical value as a score for each feature correspondence, we discover that there exists very different numerical distribution between true and false correspondences in terms of such value.
Grid Framework
Grid Pattern: For fast computation, we assign the matches in the same cell with shared motion statistical value, rather than calculating motion statistics for each correspondence. With multi-cell generalization, our method also contains global information to some extent.
Rotation & Scale: The grid pattern can be rotated in 8 directions and also could be fitted with a large range of scale change by varying the size of the grid.
Sample Results
Wide Baseline: Qualitative wide-baseline matching results on Strecha Dense MVS datasets.
Repetitive Structure: Experiment results of our method on ZuBuD dataset.
Quantitative Evaluation
Matching Performance: Evaluation on the standard VGG and StrechaMVS datasets and the large-scale real-world TUM SLAM sequences.
Pose Estimation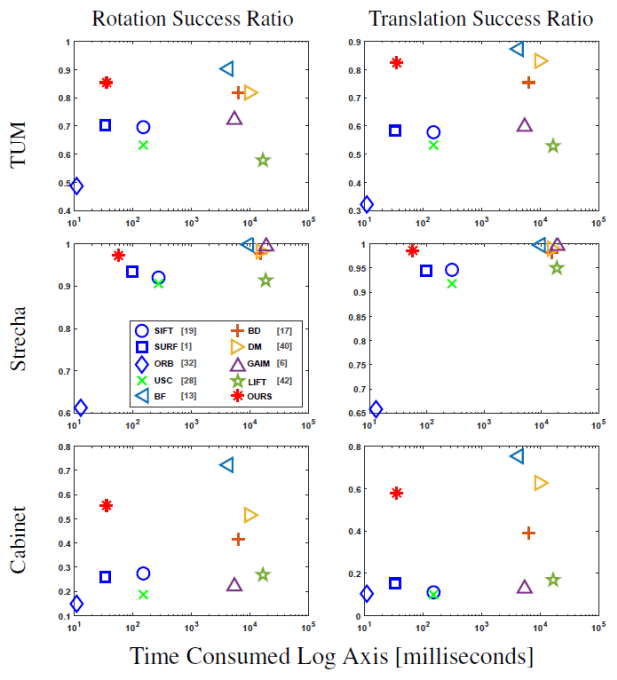
Pose Estimation: Evaluation on all the datasets with the known camera poses. The success ratio and computation time are both evaluated.
Paper Errata
Youtube Video Demo
44371total visits,55visits today
你好,论文中的假设2和公式2一直没有理解,你能再稍微深入的解释一下吗?非常感谢!
公式2说的是。如果一个匹配是错误的,那么它就有可能匹配到右边图像中任何一个特征点,因此它到达的区域是随机的。当然落在b区域中的可能性就是m/M.这里小m是b区域中特征点的个数,M是总共特征点的个数。
您的这篇文章外网打不开呢。另外,CVPR2017 给与会者发了论文集么?
不清楚你为什么打不开,你可以发email给我,然后我给你发过去pdf.
什么论文集?应该没有
文章已经获得了,拜读大作ing。我在网上没找到CVPR2017的accepted paper list,找到的只有accepted paper id list,想看看里面专业相关的论文。因为有的会议会给与会者发一个U盘里面会有accepted paper合集,所以就问问CVPR是否也有^_^
老师,您好!对于您论文中的哦你公式(3)不是太理解。如果已知a,b指向同一个区域,那么这个点匹配正确的概率不是变得大一些吗?为什么这两者是独立的?
我们是首先假设所有匹配之间是相互独立的,然后才有了后面的分析。
您好,老师!
拜读了您的论文和代码,简单有效啊。
但对文中公式(14)的阈值近似不太理解,麻烦您解释指导下,非常感谢!
非常抱歉,公式(14)上面一行有一处笔误。应该是 sf 而不是 sqrt(sf). 阈值这一块请参考代码,我之后会更正一下。
老师,
您好!
本人比较愚钝,还是不太理解sf近似等于sqrt(ni),还得麻烦您解释深入些,十分感谢啊!
sf在公式(8)中。 我们把pf(1-pf)当成一个常数提出去,所以Sf正比于sqrt(ni)。
请问,你的这个gms算法可用于3d点云的特征提取和匹配吗?
可以的。之前做过相应实验。
Hi, thanks for your contribution, seems very cool.
I read your code, you extract orb and match them by using brute force, then use your method to reject outliers.
What I want to know is, in the video, how do you match the SIFT feature and reject outlier or not?
It’s 2nn ratio test(0.66 as many previous works exploit), as the proposed method is an alternative to that.
你好~ 在我的机器上,没有跑出实时的效果,请问有可能是什么原因?
我的机器情况如下:
OS: Ubuntu 14.04 LTS
CPU: Intel® Core™ i7-6700K CPU @ 4.00GHz × 8
GPU: GeForce GTX 1080/PCIe/SSE2
OpenCV Version: 3.2.0
Cmake Verion: 3.4.1
实验设置:
输入图片: 480×720,
跑一百次平均时间(不包括划线的时间):
CPU 时间: 1.39s
GPU 时间:0.21s
多谢了~
首先你要确保是RELEASE而不是DEBUG.
另外,real time是指在视频序列上,而不是两张图片之间。(就算是两张图片也用不了这么久,2*30ms(ORB on cpu)+25(NN on GPU)+1 < 100ms)。你要确保你的opencv和cuda架构问题。 如何在视频上实时运行? 1. 视频上每次只需要计算一张图片的特征。所以ORB 30ms. 2. NN 匹配25ms可以与ORB并行。前后图片的并行。所以每一帧来的时候总时间是max(30,25) = 30ms。 3. 加上GMS的1ms总共31ms。大于30fps.
多谢回复~
我在我这边跑的时间,ORB 和NN的时间是对的,在GMS这里还有点疑问,
gms_matcher gms(kp1,img1.size(), kp2,img2.size(), matches_all); [1]
这一行代码的的时间为0.0003s,
num_inliers = gms.GetInlierMask(vbInliers, false, true); [2]
这行代码的时间为:0.1026s
这样结果是合理的吗?
你把第二个true改成false.时间还是有问题,我也查一下。
要达到您网上视频里的效果,GetInlierMask的第二,第三,两个参数,都要是false吗? 输入是多大呢?
都是false.然后用1w个点。
更新,这个时间是在cmake -DCMAKE_BUILD_TYPE=Debug 的模式下测的, GetInlierMask函数的第三参数为true的情况下测的时间。
改为 cmake -DCMAKE_BUILD_TYPE=Release ,同时GetInlierMask函数的第三参数也设为false之后,GMS的时间可以在1ms以内。
边老师,您好。昨晚听了您的报告,深受震撼,您的算法效果很好。小白我才刚刚接触这个部分,今天运行了您的代码,可是总是有错误,还望您指导一下,谢谢。 这里不能上传图片,我把错误信息写在下面。
=========================分割线===============
MEX 配置为使用 ‘Microsoft Visual C++ 2013 Professional (C)’ 以进行 C 语言编译。
警告: MATLAB C 和 Fortran API 已更改,现可支持
包含 2^32-1 个以上元素的 MATLAB 变量。不久以后,
您需要更新代码以利用
新的 API。您可以在以下网址找到相关详细信息:
http://www.mathworks.com/help/matlab/matlab_external/upgrading-mex-files-to-use-64-bit-api.html。
要选择不同的语言,请从以下选项中选择一种命令:
mex -setup C++
mex -setup FORTRAN
错误使用 mex
文件扩展名 ” 未知。
出错 Compile (line 19)
mex (‘MexGMS.cpp’, ‘D:/OutPutResult/GMS/include/’, IPath, LPath, lib1,
lib2, lib3, lib4);
出错 demo (line 4)
Compile;
===================结束线======================
我怀疑是mex()里的第二次参数路径有问题,可是自己试了几次,还是不对,不知道怎么改了。
注意 ‘-I../include/’。
在你的path前要写上’-I’,中间不要加空格。如:‘-ID:/OutPutResult/GMS/include/’.
这个是在我mac上跑的结果,特征点10k,参数没有改动:
ORB took: 123.09ms
BF took: 2613.63ms
GMS took: 12.702 ms
Get total 616 matches.
Totally took: 2749.64ms
可以看出的是BFmatch消耗了绝大部分的时间,而且比sift的时间要长很多;而且surf对于这两张狗狗好像是不match的
匹配时间需要统计多次匹配取平均,细节参照之前的回复。
老师,您好。最近在拜读您的论文,对于假设3不是很理解,您能稍微解释一下这个假设吗?谢谢。
就是说motion smoothness通常不是只存在于很小一个区域,他相连的区域也可能是连续的。
边老师,你好
我第一次用mex这个命令 不太熟悉 这个是说我缺少那几个库吗?
=======================================================================================================
>> Compile
MEX 配置为使用 ‘MinGW64 Compiler (C)’ 以进行 C 语言编译。
警告: MATLAB C 和 Fortran API 已更改,现可支持
包含 2^32-1 个以上元素的 MATLAB 变量。不久以后,
您需要更新代码以利用
新的 API。您可以在以下网址找到相关详细信息:
http://www.mathworks.com/help/matlab/matlab_external/upgrading-mex-files-to-use-64-bit-api.html。
要选择不同的语言,请从以下选项中选择一种命令:
mex -setup C++
mex -setup FORTRAN
错误使用 mex
MEX 找不到使用 -l 选项指定的库 ‘opencv_core320’。
MEX 查找具有以下名称之一的文件:
libopencv_core320.lib
opencv_core320.lib
请使用 -L 选项指定此库的路径。
出错 Compile (line 19)
mex (‘MexGMS.cpp’, ‘-I../include/’, IPath, LPath, lib1, lib2, lib3, lib4);
手动编译的话需要这几个库。如果是直接下载的opencv。只要链接opencv_world这一个库就行了
你好,我也是一样的问题,请问你后面怎么解决的
老师您好,我运行程序到orb->detectAndCompute()时就会报错,提示:
Windows已在test.exe中触发一个断点。
其原因可能是堆被损坏,这说明test.exe中或它所加载的任何DLL中有Bug。
原因也可能是用户在test.exe具有焦点时按下了F12。
希望老师能帮助解决一下,谢谢您~
你要么重新安装一下opencv试试。这是opencv的内部函数。
边老师,请问该算法对纹理很少场景匹配效果如何呢?
比之前的算法好很多。Video(TUM)里的那个柜子就是很严重的缺少纹理。SIFT只能匹配到很少,GMS能匹配得很稳。
您好,开始使用orb提取特征点分布十分不均匀,在特征点较少的区域使用您设定的阈值会把正确匹配也删除掉,这样合理吗?
关于特征不均匀的问题,你可以试试用ORBSLAM中的ORB特征。或者直接增加特征数量使它充满整张图
老师你好,请问视频的大小,分辨率有什么要求吗?
分辨率越高,效果越好,越慢。
你好,能把你上次直播的ppt共享在project主页,供我们看看么?
好的,马上上传。
边老师你好,你的代码我运行出来了,我想拿GMS与LIFT做一下对比,但是LIFT运行./run.sh的时候显示错误
Traceback (most recent call last):
File “compute_detector.py”, line 43, in
from Utils.custom_types import paramGroup, paramStruct, pathConfig
File “/home/ynh/下载/LIFT/LIFT-master/python-code/Utils/custom_types.py”, line 45, in
from flufl.lock import Lock
ImportError: No module named flufl.lock
Traceback (most recent call last):
File “compute_orientation.py”, line 40, in
from Utils.custom_types import paramGroup, paramStruct, pathConfig
File “/home/ynh/下载/LIFT/LIFT-master/python-code/Utils/custom_types.py”, line 45, in
from flufl.lock import Lock
ImportError: No module named flufl.lock
Traceback (most recent call last):
File “compute_descriptor.py”, line 41, in
from Utils.custom_types import paramGroup, paramStruct, pathConfig
File “/home/ynh/下载/LIFT/LIFT-master/python-code/Utils/custom_types.py”, line 45, in
from flufl.lock import Lock
ImportError: No module named flufl.lock
在这一步之前,我都没有出错,我刚开始已经没有安装好flufl.lock,我又pip3 install flufl.lock ,但还是这个错误
您跑出了LIFT,能不能给个经验
要不你重新安装一下吧。。我当时就照着他的README一步一步来的。
老师您使用的python2 还是python3,
python3. 代码是一个老外帮忙写的,有什么问题去github上面提交issue
你好,
我在跑LIFT的时候遇到了和你一样的问题,pip3 install flufl.lock之后还是会有“ImportError: No module named flufl.lock”错误。请问你后来解决了吗?是怎么解决的?
您好,您C++代码里这个max_inlier变量指的什么呢,它的值是由gms_header.h中413行int num_inlier = sum(mvbInlierMask)[0]赋予的,没看太懂,麻烦解释下,谢谢!
跑多尺度或者多旋转的时候每种情况下都会出来一组inliers.然后我们要挑哪种情况下出来的最多。就当作最终结果。max就是最多的意思。
边老师,我尝试用自己拍摄图片把GMS与ORB,SURF,SIFT比较了下,效果挺不错的。但还是有两个问题(1)出现了当在空间上旋转角度比较大时有一个都匹配不上的情况(传统算法能匹配上)(2)有时候匹配错误密集在一个区域里面。
1.GMS默认是没有rotation和scale变化的。你开启就可以了,看getinliers里的后两个参数。
2.gms的原理就是发现最集中的匹配。因为那些匹配是正确的概率非常高。错误情况虽然少但也有可能出现。因为这个错误匹配的比例不会太高,所以加个ransac就可以踢掉。
您好,能解释下公式9的含义吗,还有就是怎么得来的,非常感谢。
就是量化两个分布的差异。越大表示两个分布越不同。
T = mf + (a)* sf = K* n* pf + a* 根号(K*n*pf*(1-pf))约等于 a *sf 约等于 a * 根号(n) 学哥这是为啥,有点不太理解
mf很小,可以忽略。a 是个参数,所以根号里除了n是变量以外,其他常数都可以提出来算到a里去。
老师你好通过如下函数初始化后,得到的东西是什么,我debug一个一个跑了,就是这个初始化,是用来干嘛的,里面的值在最后的
thresh = THRESH_FACTOR * sqrt(thresh / numpair);
这行代码用上了,我已经完全蒙圈了,求老师解答,谢谢老师
他们的值分别为【-1,-1,-1 【-1,-1,-1 【-1,-1,-1
0, 1, 2 1, 2, 3 2, 3, 4 。。。。。。
20,21, 22】 21,22, 23】 22,23, 24】
vector GetNB9(const int idx, const Size& GridSize) {
vector NB9(9, -1);
int idx_x = idx % GridSize.width;
int idx_y = idx / GridSize.width;
for (int yi = -1; yi <= 1; yi++)
{
for (int xi = -1; xi <= 1; xi++)
{
int idx_xx = idx_x + xi;
int idx_yy = idx_y + yi;
if (idx_xx = GridSize.width || idx_yy = GridSize.height)
continue;
NB9[xi + 4 + yi * 3] = idx_xx + idx_yy * GridSize.width;
}
}
return NB9;
}
GetNB9就是获取一个格子它周围的九宫格
老师您好,看了您的论文也跑了您的程序,觉得很不错。看到您在文章中说在pose estimation 中会很有效,不禁为之一振,因为我自己就是做slam的。有个问题想问您,现在主流的slam都是特征匹配之后用RANSAC去除outliers,那您觉得GMS是完全可以取代RANSAC还是要和RANSAC结合起来更好,比如特征匹配之后先用GMS,然后在用RANSAC去进一步去除outliers。
要结合的,gms是给ransac提供输入用来算pose的,因为gms提供的匹配错误比较少,所以ransac也能很快收敛。
边同学,你好,请问你的实验是在什么配置的机器上跑的?我看论文上没说明
普通台式机。i7-4790 和 gtx980.
老师,你好!我现在做sfm,看了您的论文也跑了您的程序,但在跑程序中,对于输入图像缩放,好像稍微大一点就不行的;我是在opencv 2.4.10版本下跑的,做了小修改,另外我想得到匹配点对保存在vector中,添加了两行代码,如下,
for (size_t i = 0; i < vbInliers.size(); ++i)
{
if (vbInliers[i] == true)
{
matches_gms.push_back(matches_all[i]);
ptsL.push_back(kp1[matches_all[i].trainIdx].pt);//保存匹配点
ptsR.push_back(kp2[matches_all[i].queryIdx].pt);
cnt++;
//cout<<cnt<<endl;
}
}
但就是不行,不知为何?
另外为了进一步剔除错误匹配点,要结合RANSAC来做,那么是计算F矩阵好呢还是H矩阵好呢?
左边是queryidx.右边是trainidx.我一般用opencv finEssentialmatrix。
同学你好,我现在也在做SFM,请问你用GMS做的效果怎么样,能不能分享交流一下,多谢!
前辈,你好,我现在也在做SFM想用下边老师的GMS,想问下您效果怎么样。现在做的没有头绪,您方便的话能不能给我指点指点,多谢多谢!
我的邮箱是 l645083398@stu.xjtu.edu.cn 如果您能看到,希望您能指导一下我,多谢啦!
关于matching部分方法选择你可以参考matchbench。我的另一个项目。新的论文暂时还没有放出来。代码和数据已经在GITHUB上了。
没想到老师这么及时回复我的信息,真是感谢,我现在就去拜读一下您matchbench的项目,多谢啦!
你好,边同学,我在Ubuntu14.04 LTS下 Intel core i7-5557U cpu @3.10GHz*4 下运行得到的时间如下:
ORB+BF+GMS=80.08ms+514ms+4.9ms,时间还是蛮大的,想问问是因为我没用release版本运行?Ubuntu怎么用release编译呢?
-DCMAKE_BUILD_TYPE=RELEASE 你测得时间应该是匹配一对图片的时候。opencv函数每次执行第一遍都比较慢。你可以连续匹配两遍测第二次的时间。笔记本上上BF的时间也应该在300以内。想达到实时,需要用gpu加速BF。GTX980能加快到25ms。
老师您好,我想问一下这个代码里面的orb算法是否可以换成sift算法,在不用GPU的情况下
最好不要。因为gms特别依赖特征点的数量。sift通常数量少,结合gms结果不好。如果在大图片上sift可以检测到非常多点的时候,用gms也是不错的。
老师,这里面的ORB是否可以换成akze算法,上面提到换成sift算法担心特征点个数问题,是不是只要特征点个数足够,orb就可以换成其他算法
对的,点越多越好。其他特征点也可以
边老师您好!最近拜读了您的论文,并运行了您给的示例程序。有个问题想请教下:在两幅图像偏移不是很大的情况下,会出现左图特征点到右图特征点匹配错误,但右图误匹配的特征点仍在正确cell中的情况(即论文公式(3)等式右边第二项)。GMS算法运行结果显示,它会将这种误匹配点给保留下来。那么是不是还需进行后续的RANSAC再筛选一遍才能得到正确的结果呢?
是的。gms只是选取好的匹配为了ransac能得到更好的结果。ransac是必需的。
Hello Mr Jiawang
Thanks for the great work and amazing paper, I used your code and it handle many situation even with lack of feature, but I have issue when image has affine transform or large rotation but in your paper it could handle that situation (i.e. figure 5 in paper).
so is there is anyway to modify the code to handle affine transformation or large rotation ??
Thanks in advance
You can change parameters in this function “int GetInlierMask(vector &vbInliers, bool WithScale = false, bool WithRotation = false)”. The default is no multi-scale and multi-rotation. orb = ORB::create(100000);”
In addition, you can boost performances by increasing feature numbers. For example, “Ptr
边老师,新年好!我看了您的GMS论文,从中很是受用,但对于论文中所述的{a,b}区域不是很理解,我想问{a,b}是一对区域集合,或者只是一对区域。具体地问题就是:在图像I1和I2中通过暴力匹配找到10000对粗匹配,而a是指10000中”某一个特征点“在I1上的邻域,还是值10000个特征点在I1上所有邻域的集合?谢谢边老师
a是指左边图像中的某一个格子。b指右边图像中的某一个格子。
边老师,您好
ppt中的公式(14)我不能理解,在设定阈值的时候,为什么sf的取值为a*sqrt(n),而不是a*sqrt(kn),这里kn为这k个region中特征的总数
这里的n是指9个格式的特征总数(和你理解的kn是一样的)。已经在Errata里面提过了。
你好,请问之前的直播视频,在哪里可以看到,谢谢了。
https://www.bilibili.com/video/av11450426/
您的算法我用Graffiti数据集,进行了验证,效果还是不错的,但是那第一张图片和第二、三、四进行匹配效果还是很好的,但是与第五匹配,如果是10000特征点,是匹配不出效果的,我将特征点总数改成更大的数字,可以达到匹配,效果一般,与第六章图片匹配,几乎匹配不出来。请问是不是对大尺度的视点变换和尺度旋转变换的效果并不是很理想?
是的。当图像变换特别大时,正确的匹配数量太少所以也很难被发现。我记的匹配1to6时如果用sift,2000+个匹配中正确的数量都不到20个。这种情况就没有办法。
边老师您好,拜读了您的论文,运行了您给的示例程序对图像效果很好,但是将帧图像转换为视频处理的很慢而且效果不理想,请问像您在ppt中展示的效果,您在这一部分是怎么处理的?
brute-force matching要用gpu。然后和cpu-based feature extraction并行。结果是可以实时的。
边老师你好,请问论文中提及到的尺寸和旋转,需要人为确定吗?
是的。我默认提供的旋转已经包含了所有方向。然后尺度足够应付大部分情况了。你也可以改得更大,如果需要的话。